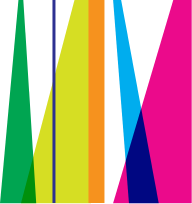
Publications scientifiques
-
11/25/2020 High risk fibrosis score prediction using computed tomography imaging
Poster presented at the Digital International Liver Congress – EASL – August 27-29, 2020 Hepatic fibrosis diagnosis is important for risk stratification, prognosis evaluation and monitoring of treatment response. Using computer tomography (CT) perfusion imaging and splenic radiomics, the objective of this study is to validate the use this non-invasive fibrosis scoring method to identify patients whose tumors are at high risk of recurrence after hepatic resection.Téléchargement 202008xx_EASL2020_Poster.pdf
Elton Rexhepaj [1], Corinne Ramos [1], Yan Liu [1], Benoit Huet [1], Olivier Lucidarme [2], Nozha Boujemaa [1] – Affiliations: [1] Median Technologies, Valbonne, France – [2] Hôpital Universitaire Pitié-Salpêtrière-APHP, Paris, France -
11/25/2020 Blinded independent central review of oncology trials: the monitoring of reader performance
Poster presented at the ESMO Asia meeting – November 20-22, 2020 The poster presents results from a study dealing with inter-reader discrepancies in image-based oncology trials in BICR settings. Inter-reader discrepancy is a metric of choice for detecting quality issues in clinical trials. The study provides reference values metrics to monitor reader performance with double read plus adjudication in clinical trials using RECIST 1.1.Téléchargement 20201120_ESMOAsia2020.pdf
Hubert Beaumont [1], Antoine Iannessi [1], Jennifer Cillario [1], Yan Liu [1] – Affiliations: [1] Median Technologies, Valbonne, France -
11/20/2020 A Deep Imaging Phenomics (DeeIP) Ecosystem to Accelerate Drug Development for Patients with Hepatocellular Carcinoma
Poster presented at The Liver Meeting, organized by the American Association for the Study of Liver Diseases, Nov 13-16, 2020 – Virtual experience Based on a meta-analysis, the poster analyses radiomics contribution to liver cancer recurrence prediction and shows how radiomics are approaching their limits for predicting disease outcome. Imaging phenomics, an emerging technology implemented in Median’s iBiopsy® platform may provide the framework to explore new associations between phenotypes which are currently not feasible using traditional radiomic workflows.Téléchargement 20201113_AASLD_Poster.pdf
Yan Liu [1] [2] , Elton Rexhepaj [3] , Afef Baili Laya [3] , Corinne Ramos [3] , Hubert Beaumont [1] , Nozha Boujemaa [3] , Anthony Lockett [2] – Affiliations [1] Medical Affairs, Median Technologies, Valbonne, France, [2] Bioscience , King’s College London, London, UK – [3] iBiopsy® Business Unit, Median Technologies, Valbonne, France -
09/18/2020 Mis-selection of non-malignant lesions as target lesions: Misclassification of RECIST 1.1 and Early Termination of Promising Drugs?
Poster presented at the ESMO 2020 Virtual Congress | September 17, 2020 |Poster Display Session 1936P For tumor response assessment in oncology trials with radiology, the baseline (BL) evaluation is critical as the selection of target lesions (TL) determines the quality of follow-up. The RECIST workgroup provided a method and recommendations for: 1) selecting TL and non-target lesions (NTL) for reporting disease evolution, 2) choosing up to 5 targets, with a maximum of two per organs. In the practice, the selection of TL is subjective; non-malignant (NM) lesions might be mistaken as TLs. The objectives of the study are 1) to analyze the impact of selecting non-malignant lesions as target lesions at baseline, and 2) to present recommendations to mitigate risks in clinical trials.Téléchargement 20200917_ESMO2020_iCRO.pdf
Antoine Iannessi [1], Hubert Beaumont [1], Yan Liu [1], Anne Sophie Bertrand [2] – Affiliations: [1] Median Technologies, Valbonne, France, [2] Polyclinique Les Fleurs, 83190 Ollioules, France -
04/08/2020 Mask uncertainty regularization to improve machine learning based medical image segmentation
Poster presented at the IEEE International Symposium on Biomedical Imaging (ISBI) | April 3-7, 2020 | Virtual conference. Segmentation of the different body structures on CT and MRI scans remains a challenging problem that requires accurate ground truth (GT) segmentation. One of the important aspects is the lack of reliability caused by radiologists annotation disagreement coupled with insufficient quality of the medical images. An independent multiple annotation is needed to overcome frequent disagreements between radiologists decisions mostly on the organs border, which is always blurred and affects on the providing an accurate segmentaKon even on contrasted CT/MRI images. Augmentation techniques aim at increasing model generalization by manipulating inputs in order to enrich set of the variability of images in regards to their GT. We introduce a method to regularize the models learning process by augmenting the information only in the associated GT masks.Téléchargement 20200407_ISBI2020_Median.pdf
Vladimir Groza [1], Benoit Huet [1], Nozha Boujemaa [1] – Affiliations : [1] Median Technologies, Valbonne, France