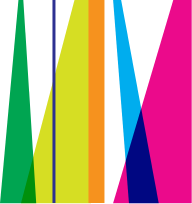
Scientific publications
-
03/28/2025 AI/ML-based lung cancer detection and characterization for lung cancer screening: results from the REALITY study on early-stage lung cancer
R. Osarogiagbon, A. Vachani, C. Gotera, l. Seijo, G. Bastarrika, E. Ostrin, J. Dennison, C. Voyton, P. Baudot, E. Geremia, P. Siot, V. Le, renoust, B. Huet, V. Bourdes -
02/24/2025 Radiomics-Based Prediction of Treatment Response to TRuC-T Cell Therapy in Patients with Mesothelioma: A Pilot Study
H. Beaumont[1], A. Iannessi[1], A. Thinnes [1], S. Jacques[1], A. Quintás-Cardama [2], – Affiliations: [1] Median Technologies, Valbonne, France; [2] TCR2 Therapeutics, Cambridge, MA, USA. -
12/20/2024 Assessing immunotherapy response: going beyond RECIST by integrating early tumor growth kinetics
This study introduces an innovative way to predict clinical outcomes in non-small cell lung cancer (NSCLC) patients receiving immunotherapy by modeling early tumor growth dynamics with the Gompertz model alongside RECIST 1.1 criteria.
M. Felfli [1], A. Thinnes [1], S. Jacques [1], Y. Liu [1], A. Iannessi [1,2] – Affiliations: [1] Median Technologies, Valbonne, France. [2] Centre Antoine Lacassagne, Nice, France. -
10/24/2024 The ins and outs of errors in oncology imaging: the DAC framework for radiologists
In oncology, the seriousness of the disease amplifies the visibility of radiological errors, leading to both significant individual consequences and broader public health concerns. By leveraging quantitative approaches, the authors reframe the diagnostic process in radiology as a classification problem, a perspective aligned with recent neurocognitive theories on decision-making errors.
This structured model offers a practical framework for conducting root cause analysis of diagnostic errors in radiology and developing effective risk-management strategies.
A. Ianessi, H. Beaumont, C. Aguillera, F. Nicol, A-S. Bertrand. -
08/22/2024 RECIST 1.1 assessments variability: a systematic pictorial review of blinded double reads
The article reviews the variability in radiologic oncology assessments, particularly focusing on RECIST 1.1 criteria, which standardize evaluations to improve consistency and accuracy. It discusses how variability arises from factors like radiologist expertise, image quality, and lesion selection, and emphasizes the importance of standardized protocols and training to mitigate these issues. By addressing the root causes of variability, the article aims to enhance the precision of response assessments, ultimately leading to better patient care and clinical outcomes.
A. Ianessi, H. Beaumont, C. Ojango, A-S. Bertrand, Y. Liu