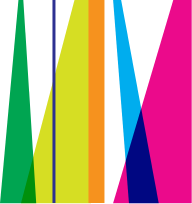
Scientific publications
-
06/05/2024 Developing a novel computer-aided diagnostic technique based on deep learning and CT images for early HCC diagnosis
Hepatocellular carcinoma (HCC) constitutes a prominent global health challenge. According to the American Association for the Study of Liver Diseases (AASLD) guideline HCC can be diagnosed by imaging examination. However, it shows that contrast-enhanced CT has limited accuracy in the diagnosis of HCC, particularly, small-size HCC lesions (≤ 20 mm) are the most difficult to identify with CT demonstrating sensitivity = 64% [1]. An Artificial Intelligence (AI) algorithm that can analyze liver CT images and localize HCC lesions would be valuable for patients at risk of HCC. Recent studies have presented promising outcomes regarding the application of AI algorithms in HCC diagnosis; However, the efficacy in localizing small-sized HCC lesions in CT images remains uncertain, and there is a need for improvement in reducing the false-positive rate [2-4].
O. Lucidarme, V. Paradis, C. Guettier, I. Brocheriou, J. Shen, S. Poullot, V. K. LE, V. Vilgrain, M. Lewin-Zeitoun -
05/24/2024 Double reading performance and the impact of adjudication on progression-free survival estimations: Findings from a lung clinical trial
The FDA recommends Blinded Independent Central Review (BICR) with double reads for imaging in clinical trials, but inter-reader variability raises concerns. Our study examined this variability in lung clinical trials using RECIST. We analyzed 5 phase III trials with 7 readers forming 11 teams, covering 1,017 patients. The study focused on Discrepancy Rate (DR), bias, endorsement rate, and the impact of adjudication on Progression-Free Survival (PFS). Results showed significant bias among readers, affecting double readings but no correlation between bias and DR. Additionally, adjudication significantly affects PFS. These outcomes highlight the need to improve monitoring in clinical trials.
Hubert Beaumont, Antoine Iannessi; Median Technologies, Valbonne, France -
03/13/2024 Unraveling Immune Therapy Efficacy Through Growth Kinetics Modeling: A Descriptive Analysis of Imaging Kinetic Biomarkers Using RECIST 1.1 Assessments
Antoine Iannessi [1], – Affiliations: [1] Median Technologies, Valbonne, France. -
12/05/2023 End-to-End AI model for Nodule Detection and Characterization in Lung Cancer Screening: Performances and Subpopulation Analysis
The abstract presents the performances and subpopulation analysis of a computer-aided detection and characterization (CADe/CADx) AI model developed to aid lung cancer screening standard of care. AI 3D-CNN models were used on a test set containing 136 cancer and 2,027 benign patients. Performance at nodule level in terms of AUC-ROC is 0.987, significantly outperforming the NLST Brock model (AUC-ROC 0.971). This result is also consistent across nodules’ key characteristics (size, attenuation, margin) and shows it could aid clinicians in optimizing their clinical routine and the clinical management of patients.
P. BAUDOT [1], C. VOYTON [1], G. DE BIE [1], V. LE1 [1], D. FRANCIS [1], E. GEREMIA [1], B. RENOUST [1], P. SIOT [1], Y. LIU [1], B. HUET [1] – Affiliations: [1] Median Technologies, 1800 Route des Crêtes, 06560 Valbonne, France. -
12/05/2023 A CT Imaging Biomarker for CD8+ Lymphocytes Infiltration Stratification in Patients with Non-Small Cell Lung Cancer
The aim of the study is to investigate the ability of radiomics features to stratify patients based on CD8+ lymphocyte infiltration levels and identify relevant radiomics features associated with these levels. The data used in this study originates from two open-source repositories, TCIA and TCGA. The AI model was trained using TCIA data and tested on TCGA data. The results suggest that four texture features can confidently discriminate CD8+ infiltration levels (high/low). The model achieved a mean area under the curve AUC-ROC of 0.73(±0.08 std) on the training set and an AUC-ROC of 0.67 (95% CI: 53%, 80%) on the test set.
F. Zerka [1], M. Felfli [1], C. Voyton [1], A. Thinnes [1], Jacques [1], Y. Liu [1], A. Iannessi [1,2] – Affiliations: [1] Median Technologies, Valbonne, France. [2] Centre Antoine Lacassagne, Nice, France.